In the realm of technology, intelligence refers to the ability of systems to emulate human-like decision-making, especially when confronted with novel and unforeseen situations. Achieving this level of capability requires leveraging paradigms like learning and inference, which are at the heart of intelligent software development.
The Evolution of Intelligent Systems
The journey of inferential learning began long before the prominence of neural networks. In the 1970s, expert systems were designed to address challenges in diagnostics and other specialized fields. These systems utilized first-order logic and inference to derive unseen rules and relationships from a limited set of encoded domain rules. The introduction of Prolog further standardized first-order logic-based inferential learning, paving the way for more systematic approaches.
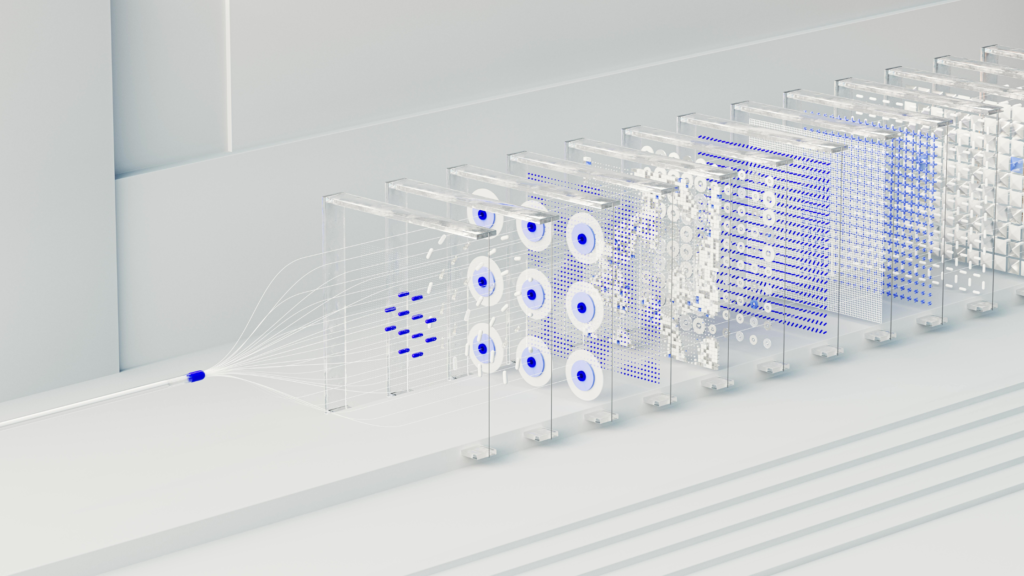
In parallel, neural networks were also gaining attention, though their journey was far from smooth. The field experienced significant setbacks during the so-called “AI Winters.” However, breakthroughs by pioneers like Geoffrey Hinton, Yann LeCun, and Yoshua Bengio revitalized interest in neural methods of learning. Innovations in deep learning combined with advancements in hardware—from Graphical Processing Units (GPUs) to modern Intelligent Processing Units (IPUs)—have enabled the replication and even surpassing of human-like decision-making capabilities. This marks the dawn of an exciting era, aptly termed the “AI Reconnaissance Age.”
Trends in Machine Learning and AI
The roots of modern machine learning can be traced back to the early 20th century when Sir Ronald Aylmer Fisher introduced statistical methods like regression and hypothesis testing. Despite the field’s advancements, there remain significant challenges and untapped opportunities. For instance, some state-of-the-art systems, initially deemed successful, have been found to exhibit biases during real-world deployment. Addressing these biases is now a critical area of research, essential for creating fairer systems with predictable outcomes.
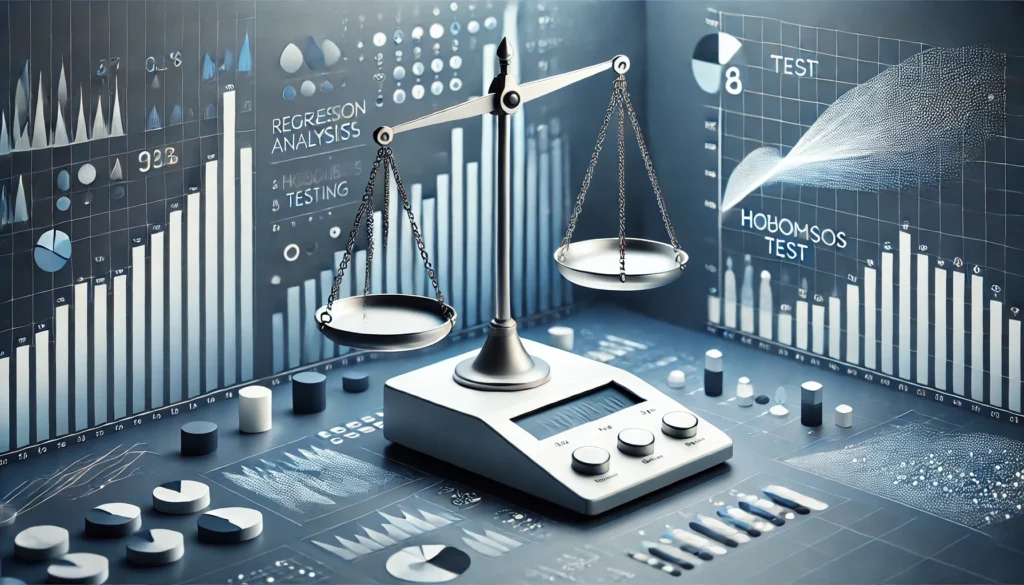
On the flip side, the adoption of AI systems across industries presents a mixed picture. While startups and niche players are rapidly leveraging AI to create specialized solutions, many traditional businesses still rely on older automated systems. This dynamic is particularly evident in sectors like FinTech, where smaller companies use AI to deliver superior user experiences, challenging incumbents in areas like insurance and investment banking.
The Role of Strategic AI Partners
For industries unable to sustain dedicated AI practices, partnerships with experienced technology providers are crucial. Companies like SB Solutions offer expertise in AI, Blockchain, and other transformative technologies, enabling businesses to adopt cutting-edge solutions efficiently and effectively.
Conclusion
As we navigate this transformative decade, the impact of deep tech on businesses and society is undeniable. By 2030, we will likely look back with awe at the strides made in leveraging technologies like AI to drive innovation and sustainability. The potential of these advancements to benefit humanity and the environment underscores the importance of embracing this change today.
Discover how SB Solutions can help you navigate this exciting journey. Let’s shape the future together!
0 Comments